“You could say it was bold. You could say it was crazy; maybe even arrogant. But I decided that if Georgia State was going to do something really big, this was the goal whose achievement would allow us to change the world.”
Mark Becker, president of Georgia State University, is reflecting on his decision, soon after taking up the position in 2009, to reword and prioritise an objective in the university’s mission statement about student retention and graduation rates.
At this point, he had absolutely no idea how he was going to achieve the objective, which stated that the university would “become a national model for undergraduate education by demonstrating that students from all backgrounds can achieve academic and career success at high rates”. Yet, within three years, Georgia State’s black, Hispanic, low-income and first-generation students were graduating at the same rate as the student body overall: a first in US higher education.
Becker achieved this major milestone thanks to the power of data analytics – or big data, as it is often termed. This involves the collection and analysis of large datasets to reveal patterns and trends. And, in universities, the approach is increasingly being used to support students, manage staff effectively and make strategic management decisions.
The US has long had a retention issue in higher education, with the high cost of courses and low levels of student preparedness being key factors. One undergraduate in three on four-year degrees does not graduate within six years, according to the US National Center for Education Statistics.
But for some groups, such as non-white students, first-generation students and those from low-income backgrounds, the statistics are even worse. For instance, only about four in 10 black students graduate within six years. Graduation rates at US institutions are therefore largely a function of the characteristics of the students they admit, with the best-performing institutions typically taking higher proportions of applicants from traditional, wealthy backgrounds.
Georgia State is a large research university but its student body is not typical of such institutions since more than half come from low-income backgrounds. “The US data for those students is appalling, and we set out to show that it didn’t need to be,” says Becker.
On investigating, Becker’s team, led by vice-provost Timothy Renick, found that too many students at Georgia State were collecting credits but not getting closer to graduation. What no one had previously spotted was that students often chose the wrong classes for their intended major, or took advanced classes too soon, obliging them to waste time and money taking extra classes to catch up or repeating those that they had failed the first time around.
The reason, the team concluded, was lack of appropriate advice – and addressing that issue struck Becker as “low-hanging fruit” on his quest to improve graduation rates. “It was then that we made the commitment to big data and the commitment to having one university-wide [student] advising corps,” he says.
This realisation coincided with the arrival of new data analytics technologies in education. And, in 2011, Georgia State became one of the first universities to bring in software vendor the Education Advisory Board. The company spent weeks on its campus, building a system that would track the thousands of decisions that students make every day and predict the likelihood of their academic success on that basis.
The company used 10 years’ worth of data on every student, grade and course at Georgia State to develop and calibrate the model, which was up and running by August 2012. Every night, the system checks more than 800 variables relating to the institution’s 30,000 undergraduates to flag up those who, according to the model, are heading for trouble.
The data include which courses students have signed up for, and their attendance records and grades. But not every variable is relevant to each student: much depends on specific course choices and behaviours. For example, getting a B- in an initial maths class may not be an issue for an English major, but it could be a sign of trouble ahead for a chemistry major. In such cases, the system automatically sends the student and their adviser an email or text asking them to meet to discuss the issue. In its first year, the system prompted more than 50,000 such meetings.
The results were immediate. “We saw fewer students dropping out and improved graduation rates within the next year,” says Becker. The average time to graduation for Georgia State students has fallen by half a semester, saving those who graduated in 2016 $15 million (£12 million) in tuition fees compared with the class of 2012. And every 1 per cent increase in student retention generates an additional $3 million return on investment for the university.
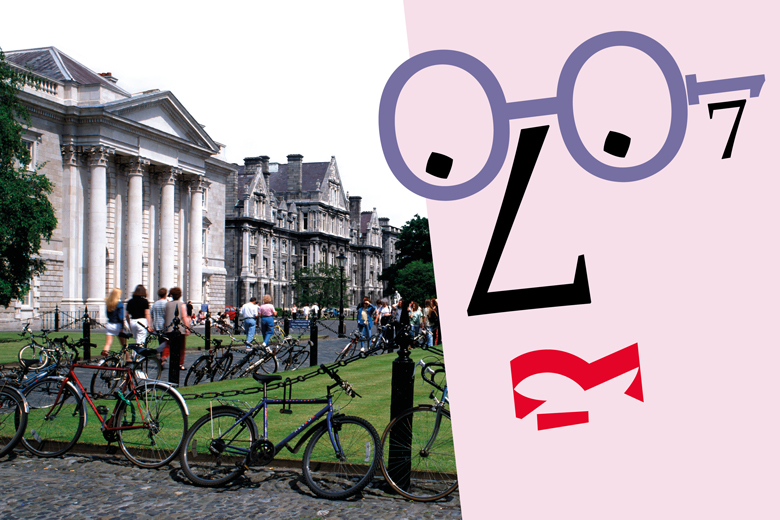
Becker says that none of this would have been possible without data analytics. Its beauty, for him, is that it allows the university to personalise the education experience for tens of thousands of students at once in an affordable way. “Our system right now is doing what professors at a very small college would do, which is keep an eye on the students. When they see something happening, [they] find a way to intervene,” he says.
Georgia State is one of 11 institutions pioneering the use of big data to boost student success rates as part of the University Innovation Alliance: a coalition of US public research universities formed in 2014 to address the need for more college-educated citizens in the US workforce. At an event at the White House in December 2014, members of the alliance declared that they would graduate an additional 68,000 students over the next decade, with predictive analytics at the heart of their plan. They are already on track to exceed that goal and graduate an extra 94,000.
Three of the UIA members, including Georgia State, were already using predictive analytics when the group was formed. These “mentor” institutions plan to help roll out proven big data strategies to assist in management decisions and academic planning across the group. Another area in which Georgia State is using big data, for instance, is enrolment. Prospective students who have accepted an offer but have yet to enrol are able to ask questions via text messages. These are answered by automated “chatbots”, which mine a database of frequently asked questions. The students “will have text message conversation[s] with non-humans and not even know it”, Becker says.
Arizona State University is another of the alliance’s mentor institutions. Art Blakemore, vice provost for academic success, says that it is using big data virtually everywhere. For example, the university has recently taken big data into the classroom in the hope of targeting one of the biggest barriers to student success: mathematics. “Many of our students were coming to [Arizona State] unprepared for college-level mathematics,” Blakemore says – adding that this problem is seen at universities throughout the country.
To help overcome it, the university abolished standard lectures on its general-level maths course and replaced them with a “mathematics emporium”, which involves students sitting at their computers and working through course content in their own time, with the help of tutors.
“It’s the exact opposite of a lecture,” says Fred Corey, vice-provost for undergraduate education at Arizona State. Before students begin, the software assesses their ability against the course learning objectives and places them at an appropriate starting point so that they do not have to revise things that they already know. They are then continually assessed as they progress through the content. “The minute the courseware detects a deficiency, it remediates them on that one particular skill…it is completely personalised and adaptive,” Blackmore says. As a result, the class’ success rate has increased from about 65 per cent to about 85 per cent.
The same approach has also been applied to other large, first-year courses, such as algebra, biology, chemistry, history and psychology, with economics next on the list. “We are concentrating on lower-division courses so that we can get students off on the right foot,” Blakemore explains.
The next stage is to get the courseware from different classes to interact so that if an engineering student is struggling in, say, a physics class because of a misunderstanding of calculus, the system can direct the student to the specific part of the calculus syllabus that they need to revise.
“It would take half an hour of office hours [for a professor] to discern that it is not the physics that is the problem but the calculus. The system can do it instantaneously,” says Blakemore.
Other areas in which Arizona State is looking at using big data include matching students with peer mentors, who have experienced similar struggles at university, and a system that brings students’ financial data into the predictive analytics tools. The university’s four-year graduation rate has increased by 19 percentage points since 2006.
It is not just public research universities that are using big data. Antony Monaco, president of Tufts University, a private institution in the Boston area, says that data analysis makes an institution much more accountable to its mission.
Tufts collects reams of data about its students and staff, often via surveys coordinated by its Office of Institutional Research and Evaluation. It uses these to measure its performance and compare how it is doing against other institutions in the US and worldwide: “If you start an initiative, you want to see if you have moved a needle,” Monaco says. “Looking at data allows you to evaluate how you are doing.”
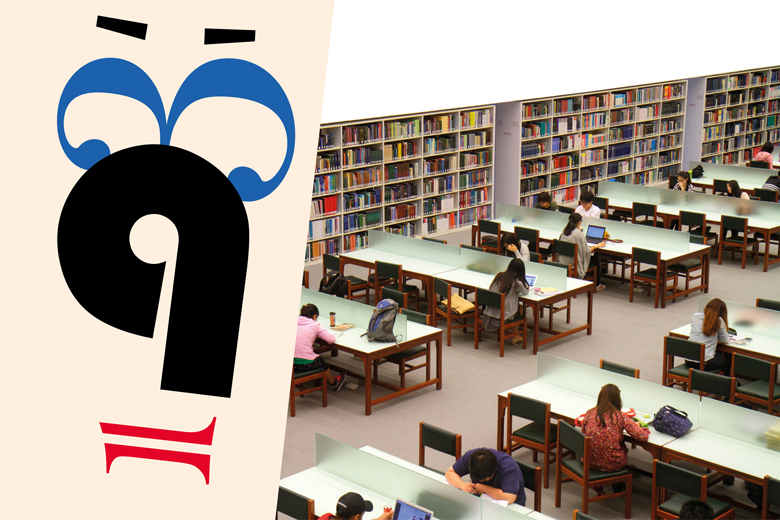
Tufts monitors the diversity of students and staff by school, for instance. It also uses analytics to track research productivity and understand exactly where research funding is spent; to carry out internal audits to flag up potential fraud; and to look at student evaluations of teaching to better understand staff performance. “Having data and dashboards to look at that helps administrators, faculty, trustees and advisers make better decisions,” he says.
But, for the most part, the use of big data in US private, as well as public, institutions has been focused around improving student retention and success rates, particularly for freshmen. This is all down to finance, according to Bill Schmarzo, chief technology officer for Dell EMC’s big data practice and an executive fellow at the University of San Francisco School of Management, where he teaches an MBA on big data.
“[Universities] spend a lot of money to recruit students to come to the school [but] they don’t realise the financial return on those students until they become second-, third- and fourth-year students,” he says.
Unlike the University Innovation Alliance, many institutions that Schmarzo works with are tight-lipped about what they are doing because they want to protect what he calls their “trade secrets”. But areas he has worked with them on include using big data to target students with a high probability of graduating, and to boost donations by better understanding the process of alumni giving.
He adds that the “holy grail” for some institutions is to tailor their offers, and even course provision and curricula, to people likely to give generous donations after graduation. “If you start doing that, then the student retention problem becomes an even bigger issue because the potential donations are leaving the school [if students drop out],” he explains.
There are, of course, ethical debates about using big data in this way. Many would baulk at the idea of using data on a student’s background to influence whether they are offered a university place. But Schmarzo counters: “There are opportunities for universities to expand their community and society roles by recommending their rejected students to other educational institutions that might be more suitable…given that student’s interests and capabilities” such as “a vocational school, junior college or local community college. You have a chance to make sure you get the right students going to the right schools, getting the right experience.”
The idea of tracking a student’s every move could also raise hackles about universities acting like Orwell’s Big Brother. But Bridget Burns, the UIA’s executive director, insists that modern students are well used to such practices.
“It is adults who seem to be squeamish about this. The reality is that this is a generation who have grown up giving a lot of their data to Facebook,” she says. Students expect the companies that they spend money with to use their information to make sure that they get the best possible service, she adds, citing the example of Amazon’s future purchase recommendations. Students “prefer not to be lost [and left] ambling without institutional support”, she concludes.
But Ben Daniel, a senior lecturer in higher education who researches big data and learning analytics at the University of Otago, in New Zealand, says that some students do have concerns about privacy and confidentiality. He believes that more research in this area is needed – although he also notes that most data in learning analytics programmes is anonymised, and that students generally provide it willingly if proper consent is obtained.
What has proved more controversial to date has been the use of big data to manage academic performance. For instance, bibliographic “metrics”, such as citation data and journal impact factors, are increasingly being used as proxies for researcher performance, reflecting their use in university rankings and national research assessments. However, such approaches remain highly controversial, with many scholars questioning how closely citations reflect the quality and importance of an individual’s research and urging managers to treat metrics with caution. Several individual institutions, including Loughborough University and the University of Bath, have responded by issuing statements on the responsible use of metrics in hiring, promotion and staff evaluation.
Critics are even more sceptical of the rise of alternative metrics, or “altmetrics”, such as the number of Twitter retweets or Facebook likes that a paper receives. Advocates suggest that altmetrics are a possible early indicator of academic impact, as well as reflecting researchers’ wider engagement or impact. However, such claims remain highly controversial.
Less contentious is a system that Jisc, the UK’s higher education technology body, is working on in partnership with 17 universities to automatically track how much money researchers are spending on open access fees when they publish their research. This collaborative, national-level approach is typical of the UK sector’s approach to big data, according to Sarah Davies, head of higher education and student experience at Jisc.
Another example is Jisc’s project with a separate group of universities and the Higher Education Statistics Agency to look at how big data can help widen participation, using the government’s National Pupil Database, which contains information on school results and levels of eligibility for free school meals. This is one of several tools being developed by Jisc and Hesa that will feed into the latter’s “Heidi Plus” business intelligence tool. Launched in 2015, this already offers users a range of data visualisations regarding students, staff, finances, estates and community interactions. It allows universities to compare things such as running costs, research income, staffing demographics and salaries.
Hesa, which has traditionally collected data from universities on an annual basis, is also aiming to do so more frequently in future, allowing benchmarking to be done in-year – not only by universities themselves but also by the government, funders and regulators.
Jisc is also working on a national learning analytics system that institutions could then plug their own systems into. As well as boosting graduation rates, the billions of data points collected by institutions as part of such a national system could potentially be an extremely valuable resource for future educational research, according to Davies.
“That is something that may revolutionise [the field] in the same way that the data available in other areas has really changed research in those disciplines,” she says.
Find out more about THE DataPoints
THE DataPoints is designed with the forward-looking and growth-minded institution in view
POSTSCRIPT:
Print headline: What do the numbers say?
Register to continue
Why register?
- Registration is free and only takes a moment
- Once registered, you can read 3 articles a month
- Sign up for our newsletter
Subscribe
Or subscribe for unlimited access to:
- Unlimited access to news, views, insights & reviews
- Digital editions
- Digital access to THE’s university and college rankings analysis
Already registered or a current subscriber? Login