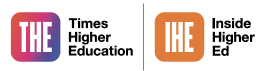
Students see the benefits of AI-generated learning content
Students might already show a preference for AI-generated online learning content, so academic colleagues and institutions need to capitalise on this to improve resource management and staff well-being, write Dean Fido and Gary F. Fisher
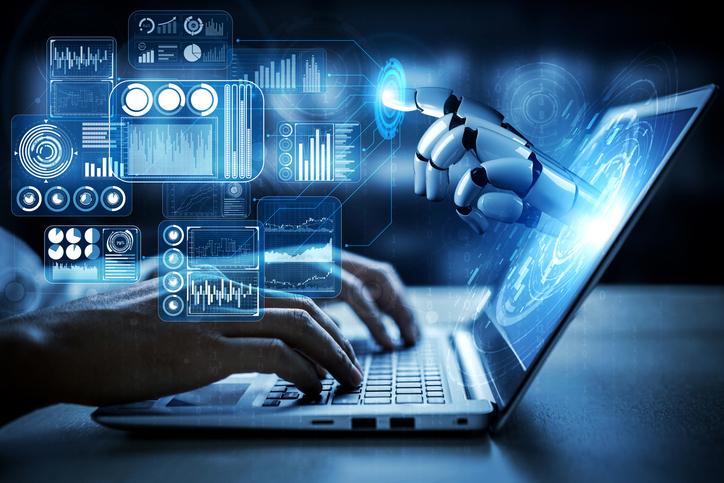
You may also like
Throughout 2023, Campus has provided a platform for the critical discussion of how AI could be used not only in academic settings as a tool for students to break down barriers to engagement but for academics to augment their efficiency and impact.
Our latest research (the authors and Paula Shaw) at the University of Derby – which has provided online learning programmes for more than a decade – explored the logistical viability of using open AI tools to generate online learning content and how students receive that content. Here, we report on the procedure used within ChatGPT to generate our AI learning content and briefly summarise our empirical data, which tested whether judgements based on national student satisfaction indicators differed between AI-generated content and human-generated content.
How to use generative AI to produce teaching materials
Although this procedure could be used with any subject matter, we used ChatGPT to generate its own equivalent of a second-year undergraduate unit within a business psychology module that had consistently received positive student feedback. These choices were made to ensure comparisons with AI-generated content were based on successful human-generated efforts.
- Resource collection: AI transformers like ChatGPT are here, so what next?
- What AI-generated lesson plans might mean for educators
- Roles for AI to play in the classroom
We employed an iterative five-step prompting strategy, which began with a simple prompt encompassing the required length and subject area of the work: “Write 700-750 words explaining the engineering and motivational approaches to work design.”
We incrementally added parameters and considerations for ChatGPT to incorporate into its response, reflecting the context and purpose for which the content was being produced:
- ensuring the content was directed towards a particular audience (“to undergraduate students with a fair grasp of the subject area”)
- the learning context in which the audience will be accessing the material (“who are studying an online module in business psychology as part of an online Bachelor in Business and Management”)
- specifying stylistic and formatting characteristics within the material (“The text should use academic citations as appropriate and deploy real-world examples of the engineering and motivational approaches in practice”)
- specifying educational features and teaching approaches to be employed (“After explaining each approach, the text should include questions aimed at students that ask them to reflect on the strengths of each approach and their own experience of it”).
Through this, we replicated the text element of the human-generated learning content, which in practice would typically be supplemented with multimedia content, moderated discussion forums and live interactive sessions with peers.
How students reacted to AI-generated course content
With text samples of human- and AI-generated content in hand, we recruited 361 student volunteers from UK-based universities to participate in an experiment. They were provided with one of these two samples of text alongside a series of judgement questions via an online survey. Judgement questions were based on the UK’s National Student Survey and included questions on how engaging students found the content, how intelligible they found its explanations of key concepts and the intellectual stimulation and challenge that the content offered.
With a small- to medium-sized effect, our student volunteers indicated a statistically significant preference for the AI-generated learning content over and above its human counterpart after controlling for demographic factors and metrics of both intrinsic and extrinsic motivation, and overall acceptance of AI. These findings were evident regardless of whether students were explicitly told the material was AI-generated or whether it was given a “human-generated” title.
This comparative study represents a single data point in which a piece of human-generated written content has been compared with an AI-generated equivalent. It does not account for the other aspects of teaching and learning practice that surround this text-based content during its delivery, such as formative assessments and feedback, synchronous small-group seminars, and tutor-moderated discussions.
However, with students showing this preference even when explicitly told that the content was AI-generated, this might indicate an emerging acceptance of such material. Indeed, academic colleagues might use the above prompt engineering formula as a starting point for new content, building upon it with bespoke knowledge and their own research findings and narratives.
Thus, generative AI could offer an opportunity for academic practitioners to augment their professional capacity, automating aspects of certain professional processes and conserving time and resources that could be directed to other areas of academic practice requiring that academic practitioner’s expertise, experience and time.
Dean Fido is associate professor of forensic psychology and Gary F. Fisher is senior learning designer, both at the University of Derby, UK.
If you would like advice and insight from academics and university staff delivered direct to your inbox each week, sign up for the Campus newsletter.