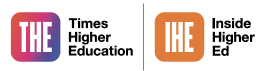
Three ways to leverage ChatGPT and other generative AI in research
A guide to three key uses of generative AI tools like ChatGPT in developing and enhancing research

You may also like
Popular resources
The barrage of resources, articles, guidelines, commentaries and webinars relating to the new wave of generative AI models such as ChatGPT, LLaMA, Bard and Sparrow is ample evidence of their potential disruptive force in higher education and elsewhere.
ChatGPT is the fastest-growing consumer application in human history, reaching 100 million active users just two months after its launch. It was not built to challenge academic integrity or redefine authentic assessment, but here we are.
Amid the challenges, there are opportunities to leverage generative AI models in the tertiary education sector. Focusing on academic research, we present three critical steps for adaptive research practice using generative AI.
What is it to know?
Any application of generative AI must begin with the acknowledgement and thorough understanding of its primary inherent limitation, that it does not know. Generative AI formulates responses to questions from human operators based on the familiarity of learned co-occurrences of billions of words, sentences, paragraphs and documents.
Human knowledge is epistemological, because we can deconstruct composite knowledge and we can understand the relationships between these elements as they exist in the real world. In contrast, ChatGPT and other generative AI models are solipsistic because they do not “know” in the way we do: they lack cognition, cannot perceive, nor can they rationalise or construct knowledge, and they are unable to substantiate the source of expression. Humans can reason and critique through logic, whereas ChatGPT’s solipsistic knowledge is strictly limited to the repetitive learning of statistical patterns and regularities from a given dataset. It assumes these limits to be the real world.
- AI transformers like ChatGPT are here, so what next?
- Effective use of machine learning to empower your research
- Teaching AI literacy: how to begin
Still, we must appreciate this new form of intelligence for its highly effective human-like conversation with seemingly meaningful responses to questions, explanation, summarisation, classification, extraction, generation, ideation, inference and rephrasing tasks.
Let us simplify academic research into three primary stages: (1) research question: determine a hypothesis or question; (2) research method: follow an accepted research method or invent a new method or algorithm to conduct an investigation that resolves or answers the question; and (3) research output: formulate, evaluate, and document the solution to enable further research.
1. Sharpen the research question
By design, ChatGPT can effectively brainstorm ideas, insights and correlations that are not immediately evident to a human domain expert. Therefore, in stage one, the manual process of identifying a research gap by reviewing literature, using search engines and seeking human expert consultations can now be supplemented with a conversation between ChatGPT and the researcher.
Taking an example from climate change research:
Prompt 1: What topics in climate science and climate change interventions have not yet explored the use of implementation science theories, models and frameworks?
Prompt 2: Based on these research gaps, propose up to 10 research questions on the topic of climate change research?
Even in situations where the research gap is known and basic versions of research questions have been formulated, ChatGPT can be leveraged to improve the readability and clarity of the research questions and even provide counter-arguments for further review of the questions and content. Some examples on how this can be done include:
Idea generation: researchers provide initial information or context for potential research questions and ChatGPT can leverage its learned knowledge and language capabilities to generate creative ideas.
Literature review: researchers can ask ChatGPT to provide an overview of a specific topic and compare different studies. It can assist in the development of a literature review by summarising the research available, highlighting key findings and identifying research gaps. Its ability to produce large amounts of information can save research time and provide comprehensive understanding of existing work.
Data exploration: ChatGPT can assist in exploring data by uncovering correlations. For example, you can prompt it to examine relationships between variables and to look for patterns that might not be obvious at first glance. Its outputs in this instance are valuable because its ability to process colossal amounts of data is useful in identifying interesting associations or unexpected occurrences.
Although ChatGPT offers valuable outputs for these research processes, human expertise, knowledge and critical thinking are essential to evaluate and refine what ChatGPT has generated. ChatGPT suggestions should be considered as a starting point for further exploration and validation.
2. Create research efficiencies
ChatGPT can be used to reduce the time to move from research ideation to implementation and validation. For example, ChatGPT can provide a summary of all research methods that are relevant to the research questions and problem domain. It can provide a general ranking of methods based on their potential effectiveness. For example:
Prompt 1: Identify appropriate research methods and related implementation science techniques that can inform the research design to answer each of these questions.
Prompt 2: Rank the identified methods using suitable metrics of effectiveness.
When applying the research method, researchers often have to read and analyse large amounts of text. Researchers can use ChatGPT to generate textual reports by summarising key findings, identifying significant insights and providing textual explanations. For example, researchers can provide a textual report to ChatGPT and it can assist by extracting key information, condensing information by rephrasing sentences, removing repetition and providing compact information.
Following the application of the research method, the data generated can be analysed, aggregated and synthesised by ChatGPT to produce meaningful reports of text and graphical visualisations. The Code Interpreter plugin for ChatGPT can pre-process datasets, generate specific types of graphical visualisation, perform statistical analyses and draw out insights and interpretations. ChatGPT can also generate code snippets or suggestions for suitable algorithms or advanced statistical analyses.
It is important to note that while generative AI can assist in creating research efficiencies, it should be treated as an AI assistant and not as a substitute for the disciplinary knowledge expert. Critical is the need for human review and validation to ensure accuracy, interpretability and relevance of the generated outputs.
3. Validate research
The human researcher translates results into findings and insights that might solve the research question or hypothesis or generate new questions. ChatGPT can be used to support these conclusions by providing further integrations of validation in several ways. For example:
Validation framework: ChatGPT can provide suggestions for appropriate validation methods, metrics or evaluation criteria.
Data validation: researchers can describe their data to ChatGPT and it can help to identify potential data quality issues, biases or limitations, and this can support researchers in resolving issues of data integrity and validity.
Method validation: ChatGPT can help to validate the methods used in your research. For example, it can provide feedback, suggest improvements or identify potential pitfalls of your research methodology, algorithms or models.
Feedback and review: research findings can be presented to ChatGPT and it can offer constructive feedback by identifying potential flaws or highlight strengths and weaknesses or areas that require further clarification.
Such integration of validation can be used to cross-check research findings so that any gaps are addressed before the research output is shared with wider audiences, such as reviewers, assessors and the general public. Generative AI can help researchers to overcome writer’s block, write simple and appealing titles for papers, construct highlights and summaries, or even a have a resourceful conversation about the topic of research.
It is timely to invest in AI as it becomes embedded in our professional and personal lives. This article advocates the case for an adaptive research practice using generative AI. Despite its versatility across the life cycle of academic research, we should always remember the tool’s inherent limitation (that it does not know) and fact-check, validate and proofread all AI-generated content.
Daswin De Silva is deputy director at the Centre for Data Analytics and Cognition, and Mona El-Ayoubi is a senior lecturer, quality and standards and educational services, both at La Trobe University.
If you would like advice and insight from academics and university staff delivered direct to your inbox each week, sign up for the Campus newsletter.