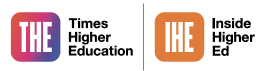
Interdisciplinarity is a core part of AI’s heritage and is entwined with its future
To train students to engage responsibly with artificial intelligence, a genuinely interdisciplinary perspective – from the language used to recognising that human and machine work in concert – is essential, write Elvin Lim and Jonathan Chase
Interdisciplinarity
Sponsored by
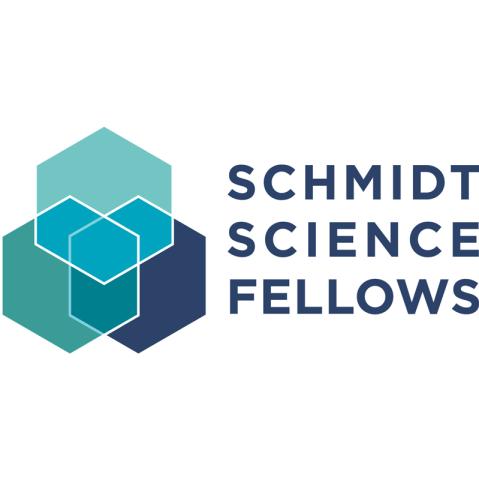
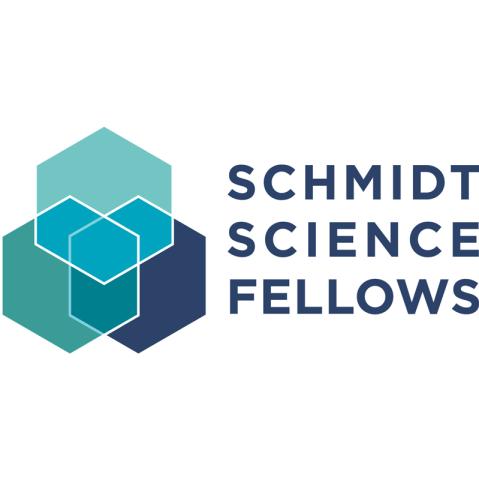
Advice for bringing together multiple academic disciplines into one project or approach, examples of interdisciplinary collaboration done well and how to put interdisciplinarity into practice in research, teaching, leadership and impact
You may also like
Popular resources
What is artificial intelligence? How we answer this question can be influenced heavily by our past exposure to AI-related ideas and stories, and the concomitant hope and fears they narrate. The emergence of generative AI tools has pushed discussion of AI and its impact to the forefront of conversation, yet many of us still have only a partial conception of what AI is, what it can do, and how to ensure it is used responsibly.
- How can we measure interdisciplinary learning?
- Collection: AI transformers like ChatGPT are here, so what next?
- ChatGPT and generative AI: 25 applications to support student engagement
This lack of clarity is a result of disciplinary fragmentation in how we approach AI, we argue. If we are to equip our students with the skills they need to truly understand AI as a technology and to embrace it as a force for positive social change, we must adopt a genuinely interdisciplinary approach to understanding and teaching AI.
The relationship between AI and interdisciplinarity
Interdisciplinarity is not a post-facto imposition on our account of AI, but an inherent part of its origins. Many of the ideas we associate with AI have their roots in the older discipline of cybernetics. Derived from the Greek, kubernētikēs – “steering” to mathematician and philosopher Norbert Wiener – cybernetics was the study of “control and communication in the animal and machine”.
By bringing together ideas from fields as diverse as philosophy, psychology, biology, sociology and mathematics, Wiener envisioned a science in which technology was developed to work in concert with humanity, based on a holistic, interdisciplinary understanding of both. Cybernetics introduced the idea of an analogy between the computer processor and the human brain, claiming that a sufficiently sophisticated computer program could act as the intelligent “brain” of the computer.
While cybernetics fell out of favour, much of its language and many of its ideas linger. Among mathematicians and behavioural and computer scientists, the focus around “intelligence” has shifted to autonomous “rational” behaviour, in which a programmed set of actions are selected based on a calculated expectation of maximised “utility”.
In the humanities, however, the analogy with a humanlike intelligent machine remained a strong theme. Futurists popularised the idea of the singularity in which machines achieved full sentience, often spelling the end of biological humanity either through transcendence or destruction. These ideas offer an effective vehicle for self-reflection – as AI serves as a mirror to humanity through which we can examine ourselves – but also describe a technology that bears little resemblance to the academic and practical reality of AI.
The real risks of AI
The problem, then, with this disciplinary divergence is a failure to appreciate that humans and technologies are continually and ineluctably in conversation and collaboration at every step of the development of AI. It becomes easy to oversimplify the risks of AI associated with sentience and replacement, presenting something of a red herring when it comes to the true risks.
The real limitations of AI lie with problems such as the way machine learning can replicate and amplify human bias, the way poorly chosen data labels can create unfair behaviours, and that organisations may place excessive trust in an AI system as objective without recognising the extent to which human decision-making, with all its merits and foibles, has governed how that data is selected, processed and acted upon.
Tackling AI risks with an interdisciplinary lens
To train students to responsibly engage with AI in society and the workplace, a genuinely interdisciplinary perspective is essential.
Make understanding AI’s possibilities accessible to all students
First, it is necessary to impart an accurate understanding of modern AI’s true nature and possibilities in a manner that is accessible to all students, not just those with the mathematical and technical expertise to take advanced-level computing classes (where AI courses typically reside).
This can come through the language we use to talk about AI. Terms such as “thinking” and “learning” can be properly recognised as analogous only when it is understood that AI is driven by mathematics formulated not to “understand” phenomena but to calculate patterns to generate accurate predictions. Arguably, AI is more “A” than “I”, and so by looking under the hood, so to speak, we can appreciate accurately how it can be used selectively to solve human problems, yet also recognise its limitations with a richness that the language of analogy does not provide.
Engage students with how human and machine work together
Second, we must revisit the core insight of cybernetics – of human and computer working in concert; we must engage not only with the mechanisms of the machine but the lived experiences of the human. It is not enough to treat AI ethics as a postscript to a technical course nor to tell students: “Don’t be evil.”
It is only by developing a deeper understanding of how humans live together, how they work and how they relate to their environment that we can find opportunities for AI to enrich the human experience. To achieve systems like this, we must look beyond the limited data of any problem at hand and leverage the expertise of other disciplines and data surrounding – and even seemingly unconnected to – the challenge to offer the best solutions. A single-track approach to machine learning without appreciation of the multifarious nature of human experience will not advance our quality of life; it will simply ensure that we repeat the mistakes of the past.
Interdisciplinarity is part of AI’s heritage and must be an integral part of its future if we wish to create a future where AI drives human flourishing. Our students must be equipped with this understanding because they will ultimately determine how AI is permitted to shape our future.
Elvin Lim is dean of the College of Integrative Studies and Jonathan Chase is assistant professor of computer science (practice), both at Singapore Management University.
If you’d like advice and insight from academics and university staff delivered direct to your inbox each week, sign up for the Campus newsletter.
Interdisciplinarity
Sponsored by
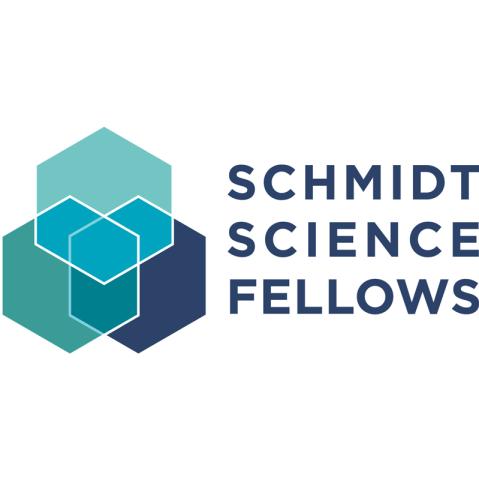
Advice for bringing together multiple academic disciplines into one project or approach, examples of interdisciplinary collaboration done well and how to put interdisciplinarity into practice in research, teaching, leadership and impact
Additional Links
For more information on this topic, see our spotlight Unpacking academic interdisciplinarity.
Interdisciplinarity
Sponsored by
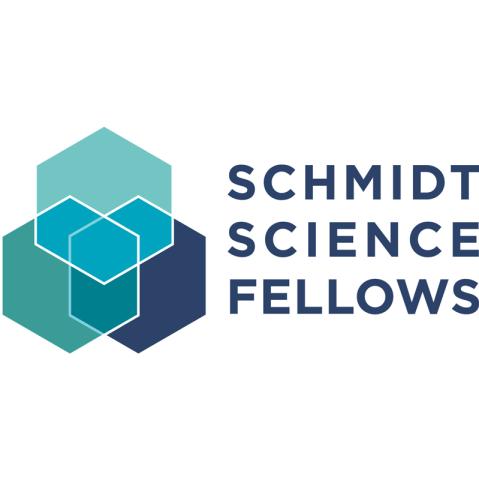