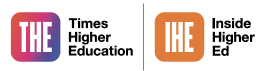
Can we spot AI-written content?
A look at common features of LLM-created writing and its implications for how we might assess students’ knowledge and skills in the future
You may also like
Popular resources
There’s a key difference between work written solely by large language models (LLMs) and co-produced work: the latter is much harder to detect because it contains human elements that vary from person to person.
Telltale signs of AI-generated text include references that do not exist, such as names of people or academic references, hallucinated by the model, along with expressions such as “As an AI language model, I am unable to…” left unintentionally by users.
LLMs, such as GPT, often structure answers in a precise, top-down manner, starting with basic definitions and an outline of the work. For instance, a colleague of mine was able to correctly deduce that a student had used GPT to write an essay because it started with concepts that were very basic for the level of the course and looked out of place. However, this isn’t always a definitive sign because weaker students might also over-explain basic concepts, sometimes to increase their word counts.
In general, I expect most students’ work to be co-produced and not entirely written by AI. Thus, the question changes from “was an LLM involved?” to “does this usage amount to misconduct?”
The downsides of using AI detection tools
I am against the use of AI detection tools. Companies train these detectors on specific datasets, but the actual uses of LLMs are so rich and varied that no dataset can fully capture the range of content that a model can produce.
At the moment, evidence suggests that available detectors are not reliable. There is also evidence that texts written by non-native English speakers are particularly vulnerable to being incorrectly flagged as AI-generated, probably because their sentences are structured more predictably.
Even if AI detectors became highly reliable, we are still shifting towards LLM co-created content for reasons such as to improve writing, save time or boost accessibility. Soon, even students’ word processors will have an integrated LLM. Using such detectors would disadvantage those who use AI for legitimate reasons. Many institutions, including governments, believe in training individuals to use AI responsibly and skilfully.
It’s intriguing to consider how assessments might evolve to be able to incorporate effective and appropriate use of AI, since we know that assessments are one of the main motivators of learning for students. Think of the analogy of calculator use in non-calculator exam papers. We do not want students to use calculators, so we create controlled conditions to prevent it, but outside of exams we acknowledge their existence and teach critical and proper use of them.
- Resource collection: AI transformers like ChatGPT are here, so what next?
- An introduction to prompting generative AI like ChatGPT for teaching and learning
- How can we teach and assess with ChatGPT?
Mitigating academic malpractice in the short term
Students have long used different ways to cheat, such as plagiarism and essay mills. LLMs only make it easier and faster. The best thing to aim for is to motivate students not to cheat by evolving assessments, eventually embracing the possibility of AI co-creation.
This is a long process, however. Mitigation in the short-term could mean measures such as:
- Assigning more in-person, invigilated assessments or oral presentations
- Asking students to submit drafts at regular intervals to see the work evolve and feedback being implemented, giving more weight to reflective elements of assessments
- Running assignments through various LLMs to get an idea of how models perform
- While mindful of privacy and accessibility, requiring students to work only within the bounds of a virtual learning environment (VLE) such as Cadmus.
A past recommendation was to add visuals in assessments because LLMs were text-limited. However, recent updates in Bard and GPT-4 now allow them to handle visual elements.
Further, it’s crucial for course leaders to define clear AI policies and guidelines, distinguishing appropriate from inappropriate use, setting standards and underscoring the consequences tied to academic misconduct.
Ensuring academic integrity in the long term
Academic staff should receive training on AI, its workings, strengths and limitations. There are damaging misconceptions such as overconfidence in the ability to identify AI-written content. Several studies reveal that humans consistently misjudge AI-written content as human-produced, especially content generated by advanced models.
Supporting workload is essential for maintaining academic integrity. Undeniably, authentic assessment requires more effort: for example, reviewing drafts and offering regular feedback takes more time than grading one essay once.
In the end, crafting more authentic assessments comes down to a human element, taking time to speak to other humans about what works. As we shift towards generative AI, enhancing scholarship and community sharing becomes vital.
GPT-4 was used for feedback and improvement of an early draft of this article.
Cesare Giulio Ardito is a lecturer in mathematics at the University of Manchester.
If you would like advice and insight from academics and university staff delivered direct to your inbox each week, sign up for the Campus newsletter.